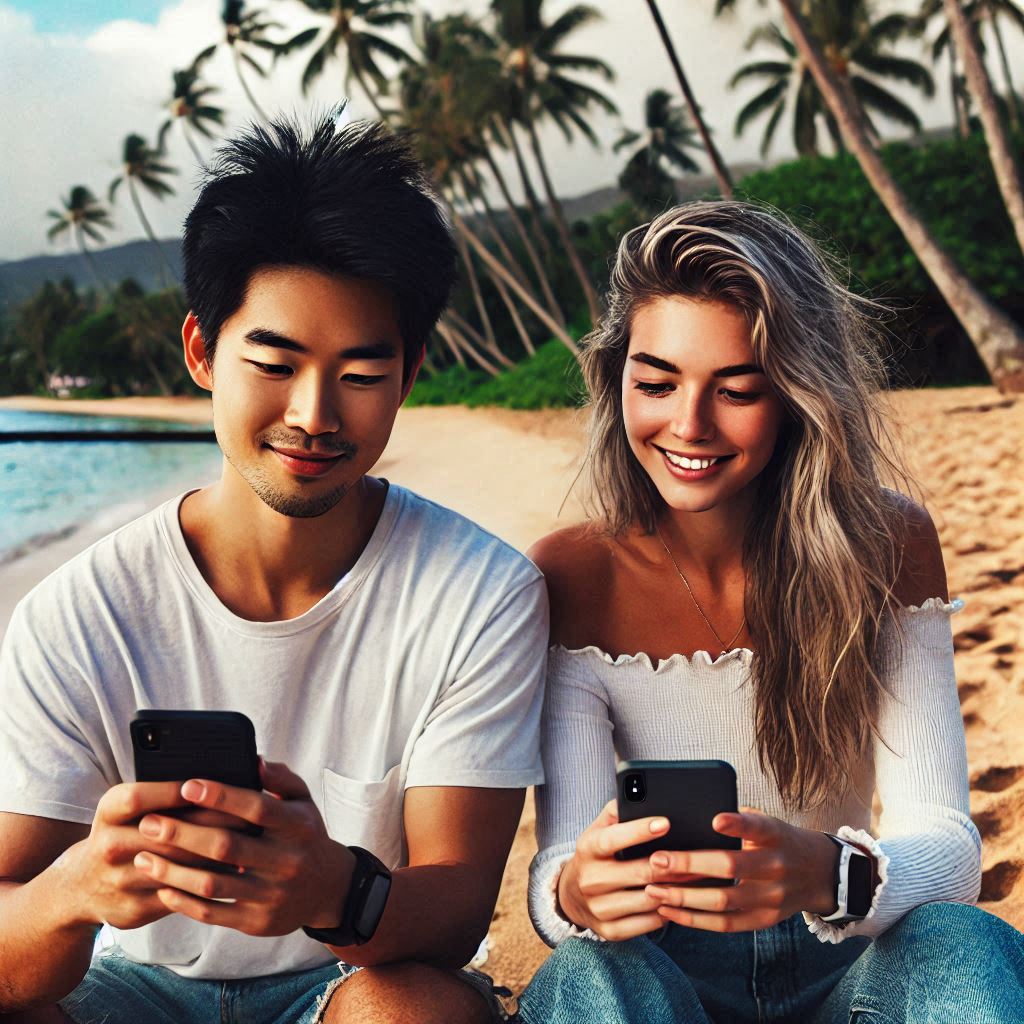
FEDS Paper: Missing Data Substitution for Enhanced Robust Filtering and Forecasting in Linear State-Space Models
Publication Date: 2025-01-03 20:45
Authors: Not specified in the provided context
Journal/Publisher: FRB
Abstract:
This paper presents a new method for handling missing data in linear state-space models by substituting missing values with imputed values. The proposed method aims to enhance the robustness of filtering and forecasting procedures, especially when the missing data pattern is irregular or complex.
The authors argue that traditional methods for handling missing data, such as listwise deletion or mean imputation, can lead to biased estimates and reduced forecast accuracy. Their proposed method addresses these limitations by incorporating a data imputation step before applying the filtering and forecasting algorithms.
Key Features of the Proposed Method:
- Imputation based on State-Space Model: The missing values are imputed using a state-space model that is fitted to the observed data. This ensures that the imputed values are consistent with the underlying dynamics of the system.
- Robust Filtering and Forecasting: The imputed values are used in the filtering and forecasting algorithms. By using imputed values that capture the system’s behavior, the filtering and forecasting procedures become more robust to missing data.
- Improved Estimation Accuracy: The paper demonstrates that the proposed method can improve the accuracy of parameter estimation compared to traditional missing data handling techniques.
- Enhanced Forecast Performance: The imputed values also lead to more accurate forecasts, even when the missing data pattern is irregular or complex.
Applications:
The proposed method is applicable to a wide range of linear state-space models, including Kalman filters, particle filters, and extended Kalman filters. It can be used in various fields, such as:
- Time Series Analysis: Imputing missing data in economic or financial time series data.
- Signal Processing: Handling missing values in sensor data or image processing.
- Econometrics: Estimating and forecasting economic models with missing data.
- Control Systems: Imputing missing values in control systems to maintain system stability.
Conclusion:
The paper presents a novel method for handling missing data in linear state-space models by using imputed values based on the underlying system dynamics. The proposed method enhances the robustness of filtering and forecasting procedures and leads to improved estimation accuracy and forecast performance. The paper provides a valuable contribution to the field of data imputation and its applications in various domains.
FEDS Paper: Missing Data Substitution for Enhanced Robust Filtering and Forecasting in Linear State-Space Models
The AI has provided us with the news.
I’ve asked Google Gemini the following question, and here’s its response.
FRB a new article on 2025-01-03 20:45 titled “FEDS Paper: Missing Data Substitution for Enhanced Robust Filtering and Forecasting in Linear State-Space Models”. Please write a detailed article on this news item, including any relevant information. Answers should be in English.
22