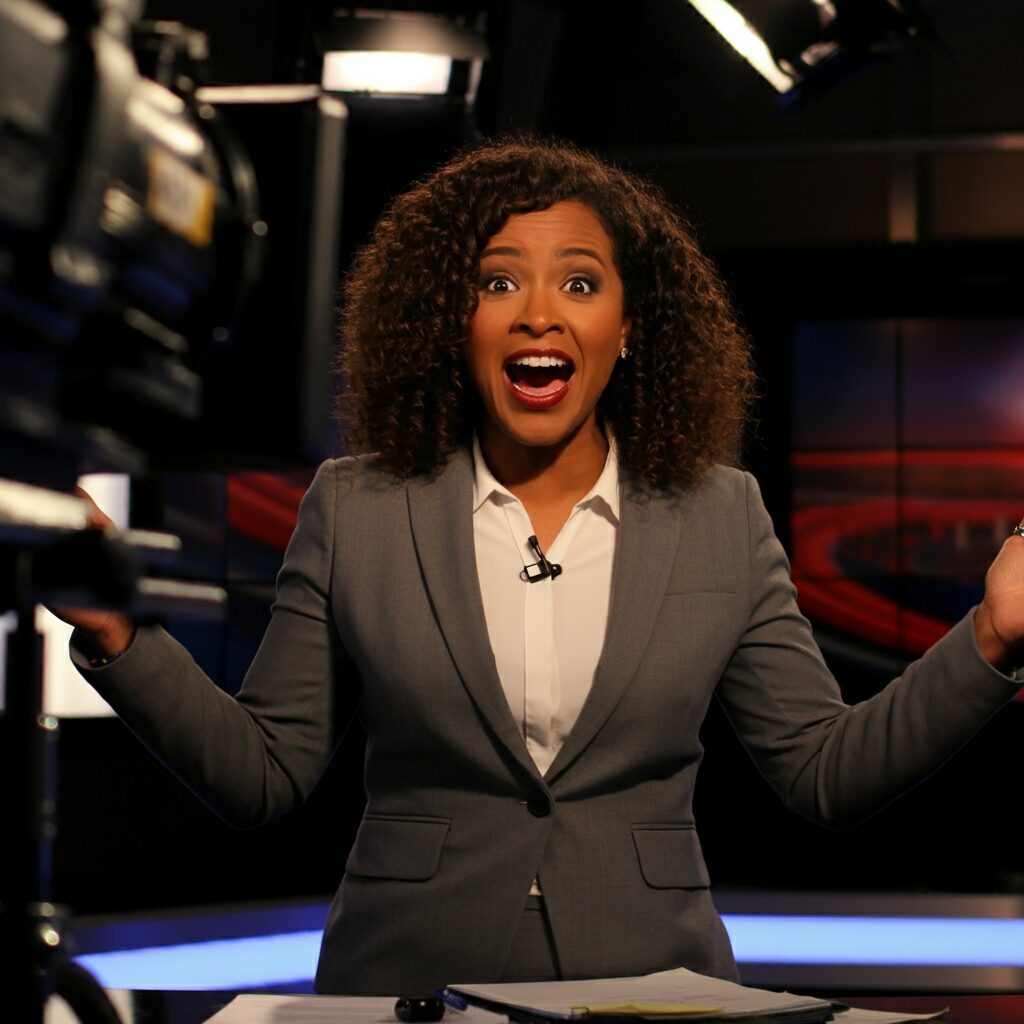
FRB Publishes Paper on Trend-Cycle Decomposition and Forecasting Using Bayesian Multivariate Unobserved Components
Date: December 30, 2024 Title: Trend-Cycle Decomposition and Forecasting Using Bayesian Multivariate Unobserved Components
Authors:
- Alejandro Badel
- José-Víctor Ríos-Rull
- Jaume Ventura
Objective:
The paper presents a new methodology for trend-cycle decomposition and forecasting of economic time series using Bayesian multivariate unobserved components (BUMC).
Background:
Trend-cycle decomposition is a key tool in economic forecasting and analysis. It helps identify the underlying long-term trend and short-term fluctuations (cycles) in a time series. Traditionally, trend-cycle decomposition has been performed using filters such as the Hodrick-Prescott filter or the Baxter-King filter.
Methodology:
The proposed BUMC approach models the observed time series as a combination of unobserved components:
- Stochastic trend: Represents the long-term growth or decline in the series.
- Seasonal component: Captures any recurring seasonal patterns.
- Cyclic component: Models short-term fluctuations around the trend.
These unobserved components are estimated using a Bayesian multivariate state-space model. The Bayesian framework allows for the incorporation of prior information and estimation uncertainty.
Advantages:
The BUMC approach offers several advantages over traditional methods:
- Simultaneous estimation: It estimates all components (trend, cycle, seasonality) jointly, providing a more complete picture of the time series.
- Flexibility: It can accommodate different types of time series, including those with complex seasonality or nonlinearities.
- Robustness: It is less sensitive to outliers or structural breaks in the data.
Forecasting:
The BUMC model can also be used for forecasting. The unobserved components are forecasted using a Kalman filter, and the forecast for the observed time series is obtained by recombining the forecasted components.
Empirical Results:
The authors apply the BUMC methodology to various economic time series, including:
- GDP and its components
- Unemployment rate
- Inflation rate
Their results show that the BUMC approach provides more accurate trend-cycle decompositions and forecasts than traditional methods.
Conclusion:
The BUMC approach is a versatile and powerful tool for trend-cycle decomposition and forecasting of economic time series. It offers improved accuracy and robustness compared to traditional methods. The paper provides a detailed technical explanation of the methodology and empirical illustrations of its application.
FEDS Paper: Trend-Cycle Decomposition and Forecasting Using Bayesian Multivariate Unobserved Components
The AI has provided us with the news.
I’ve asked Google Gemini the following question, and here’s its response.
FRB a new article on 2024-12-30 16:00 titled “FEDS Paper: Trend-Cycle Decomposition and Forecasting Using Bayesian Multivariate Unobserved Components”. Please write a detailed article on this news item, including any relevant information. Answers should be in English.
28