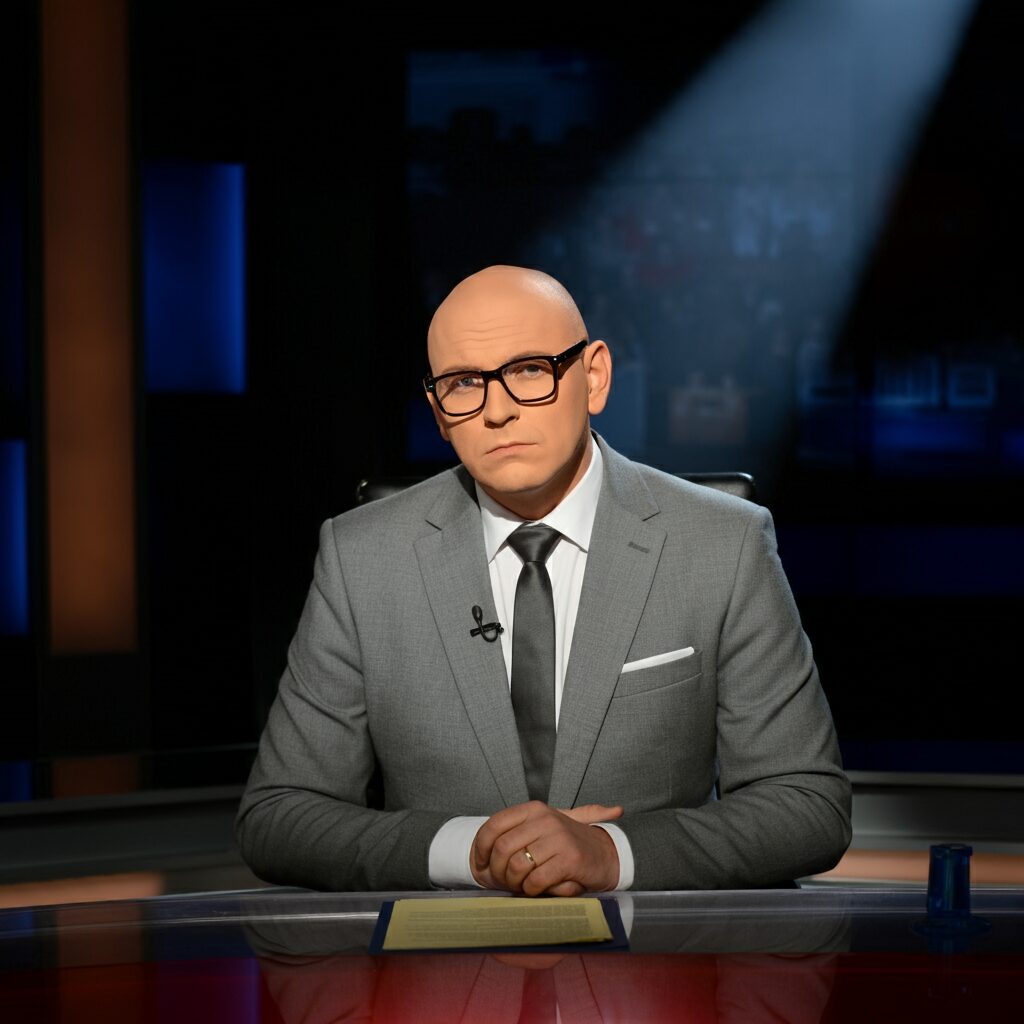
The “Watt-bit Collaboration” Strategy: Harnessing Synergy to Combat the AI-Driven Power Crisis
The rise of Generative AI (GenAI) is undeniably revolutionary, offering unprecedented capabilities across various sectors. However, this technological leap comes with a significant cost: a massive surge in electricity demand. According to a report published by the 環境イノベーション情報機構 (Environmental Innovation Information Institute) on April 18, 2025, at 2:06 AM, the solution might lie in a strategic “Watt-bit collaboration.”
The Core Problem: Generation AI’s Insatiable Power Hunger
GenAI models, like the advanced language models and image generators we’re seeing increasingly integrated into daily life, are incredibly resource-intensive. Training and running these models require immense computing power, translating directly into enormous electricity consumption. Here’s a breakdown:
- Data Centers as Power Guzzlers: The heart of GenAI resides in massive data centers packed with powerful servers. These facilities require substantial energy for computation, cooling, and infrastructure.
- Training Demands: Training a single GenAI model can consume the same amount of electricity as hundreds of households use in a year.
- Ongoing Operation: Even after training, running GenAI applications for tasks like answering questions, generating content, or controlling complex systems requires continuous power.
- Rapid Growth: As GenAI technology continues to advance and become more ubiquitous, the demand for electricity will only intensify, potentially straining existing power grids and exacerbating environmental concerns.
The “Watt-bit Collaboration” Strategy: A Two-Pronged Approach
The “Watt-bit collaboration” proposes a holistic approach to address this growing challenge by focusing on two key areas: Watt Optimization (Energy Efficiency) and Bit Optimization (Algorithmic Efficiency).
1. Watt Optimization (Energy Efficiency): Squeezing the Most Out of Every Watt
This strategy focuses on reducing the amount of energy required to power GenAI infrastructure. Key components include:
- Energy-Efficient Hardware: Developing and deploying specialized hardware like GPUs and AI accelerators designed to perform GenAI tasks with significantly lower power consumption. This includes exploring new chip architectures and advanced cooling technologies.
- Smart Data Center Management: Optimizing data center design and operation for maximum energy efficiency. This involves:
- Location, Location, Location: Siting data centers in regions with access to renewable energy sources and cooler climates to minimize cooling requirements.
- Advanced Cooling Systems: Implementing innovative cooling technologies like liquid cooling and free cooling (using outside air) to reduce energy consumption for temperature regulation.
- Smart Power Management: Utilizing AI-powered energy management systems to dynamically adjust power consumption based on workload demands and optimize energy usage across the entire data center infrastructure.
- Renewable Energy Integration: Powering data centers and GenAI operations with renewable energy sources like solar, wind, and geothermal. This not only reduces carbon emissions but also provides a more sustainable energy source for the long term.
- Carbon Capture and Storage (CCS): While controversial, CCS technologies can be implemented at power plants to mitigate carbon emissions generated from electricity production, even if the source is not renewable.
2. Bit Optimization (Algorithmic Efficiency): Making GenAI Smarter, Not Just Bigger
This strategy focuses on improving the efficiency of GenAI algorithms themselves, enabling them to achieve the same or better results with less computational power. Key components include:
- Model Compression and Distillation: Reducing the size and complexity of GenAI models without sacrificing performance. Techniques like pruning (removing unnecessary connections) and quantization (reducing the precision of numerical values) can significantly reduce computational requirements.
- Efficient Training Algorithms: Developing new and improved training algorithms that require less data and computational resources to achieve the desired accuracy. This includes exploring techniques like federated learning, which allows models to be trained on distributed data without requiring data to be centralized.
- Hardware-Aware Algorithm Design: Designing GenAI algorithms that are specifically optimized for the underlying hardware architecture. This involves understanding the strengths and weaknesses of different hardware platforms and tailoring algorithms accordingly.
- Focus on Specific Tasks: Instead of building monolithic, all-encompassing GenAI models, focusing on developing specialized models optimized for specific tasks can significantly reduce the computational burden. This allows for more efficient use of resources and avoids unnecessary complexity.
Why “Collaboration” is Key
The “Watt-bit collaboration” emphasizes the importance of collaboration between various stakeholders, including:
- AI Researchers and Developers: To focus on developing more energy-efficient algorithms and model architectures.
- Hardware Manufacturers: To innovate and produce specialized hardware optimized for GenAI with lower power consumption.
- Data Center Operators: To implement best practices for energy efficiency and renewable energy integration.
- Energy Providers: To invest in renewable energy infrastructure and explore innovative energy storage solutions.
- Government and Regulatory Bodies: To provide incentives for energy-efficient GenAI practices and promote the adoption of renewable energy.
Potential Benefits of the “Watt-bit Collaboration”
- Reduced Energy Consumption: Significantly decrease the overall energy footprint of GenAI, alleviating pressure on power grids.
- Lower Carbon Emissions: Contribute to global efforts to combat climate change by reducing reliance on fossil fuels.
- Improved Sustainability: Create a more sustainable future for AI development and deployment.
- Cost Savings: Reduce operating costs for data centers and GenAI applications.
- Increased Accessibility: Make GenAI technology more accessible to a wider range of users and organizations by reducing the resource requirements.
Challenges and Considerations
While the “Watt-bit collaboration” strategy offers a promising path forward, several challenges and considerations remain:
- Investment Requirements: Implementing energy-efficient hardware and data center infrastructure requires significant upfront investment.
- Technological Innovation: Continued research and development are crucial for developing more efficient algorithms and hardware.
- Collaboration and Coordination: Effective collaboration between different stakeholders is essential for successful implementation.
- Ethical Considerations: It is important to ensure that energy efficiency efforts do not come at the expense of fairness, transparency, and other ethical considerations.
- Monitoring and Evaluation: Regular monitoring and evaluation are needed to track progress and identify areas for improvement.
Conclusion
The “Watt-bit collaboration” strategy, as outlined by the 環境イノベーション情報機構, presents a viable and necessary path forward to address the growing power demands of Generation AI. By combining efforts to optimize both energy efficiency and algorithmic efficiency, we can unlock the transformative potential of AI while mitigating its environmental impact and ensuring a sustainable future. The urgency of this approach cannot be overstated, and collaborative action is critical to successfully navigate the energy challenges posed by the AI revolution.
“Watt-bit collaboration” strategy to overcome the power crisis in the age of generation AI
The AI has delivered the news.
The following question was used to generate the response from Google Gemini:
At 2025-04-18 02:06, ‘”Watt-bit collaboration” strategy to overcome the power crisis in the age of generation AI’ was published according to 環境イノベーション情報機構. Please write a detailed article with related information in an easy-to-understand manner.
25